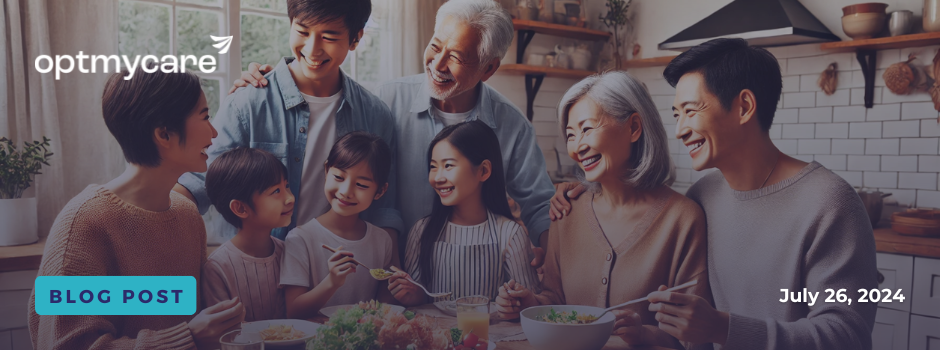
Integrating Social Determinants of Health into Predictive Analytics
- OmcEditor
- No Comments
In the realm of modern healthcare, the integration of Social Determinants of Health (SDOH) into predictive analytics is not just beneficial—it’s imperative. For healthcare providers and payers, understanding the profound impact of SDOH on patient health outcomes can significantly enhance the precision and efficacy of care delivery. This blog delves into the intricacies of SDOH, their effects on health outcomes, and the critical role they play in the future of predictive analytics.
Understanding Social Determinants of Health (SDOH)
Social Determinants of Health encompass the non-medical factors that influence health outcomes. The World Health Organization defines SDOH as the conditions in which people are born, grow, live, work, and age. These factors include:
- 1. Economic Stability: Income, employment, food security, and housing stability.
- 2. Education Access and Quality: Literacy, language, early childhood education, vocational training, and higher education.
- 3. Healthcare Access and Quality: Health coverage, provider availability, cultural competency, and quality of care.
- 4. Neighborhood and Built Environment: Housing quality, transportation, safety, parks, and playgrounds.
- 5. Social and Community Context: Social cohesion, civic participation, discrimination, and incarceration.
-
Each of these determinants interacts with others to create a complex web of influences on an individual’s health trajectory.
Impact of SDOH on Patient Health and Healthcare Outcomes
The impact of SDOH on health outcomes is well-documented. Numerous studies have demonstrated that socioeconomic factors can significantly influence health behaviors, access to care, and overall health status. For instance:
- Economic Stability:
Individuals with lower income levels often face barriers to accessing healthcare services and healthy foods, leading to higher rates of chronic conditions such as diabetes and hypertension. - Education Access and Quality:
Education is closely linked to health literacy. Lower educational attainment can result in misunderstandings of medical information, poor health management, and increased hospitalizations. - Healthcare Access and Quality:
Disparities in healthcare access can lead to delayed diagnoses and treatments, exacerbating health conditions and increasing healthcare costs. - Neighborhood and Built Environment:
Poor housing conditions and unsafe neighborhoods can contribute to stress, respiratory issues, and limited physical activity, all of which negatively impact health. - Social and Community Context:
Strong social support networks can improve mental health and adherence to medical treatments, while social isolation can have the opposite effect.
The Interplay Between Clinical Data and SDOH
While the incorporation of SDOH data into predictive analytics is a game-changer for healthcare providers and payers, it is crucial to recognize that SDOH data alone is insufficient for a comprehensive understanding of patient risk. Traditional clinical data provides a snapshot of a patient’s health status, offering essential insights into medical conditions, treatment histories, and clinical risk factors. However, without the context provided by SDOH data, it can be challenging to fully understand the factors driving health outcomes and identify the most effective interventions.
Conversely, relying solely on SDOH data lacks the clinical context necessary to determine which patients need the most help from social services. For example, knowing that a patient lives in a food desert is valuable information, but without understanding their specific health conditions—such as diabetes or hypertension—healthcare providers cannot tailor interventions effectively.
Therefore, the integration of both clinical data and SDOH into predictive models provides a more holistic and accurate view of patient risk profiles. This combined approach enables healthcare providers to identify high-risk individuals more accurately and develop targeted interventions that address both medical and social needs.
Examples of SDOH-Driven Interventions
Effective integration of SDOH into predictive analytics enables the development of targeted interventions designed to address specific social determinants. Here are some examples:
- Community Health Programs:
Initiatives such as mobile clinics, community gardens, and nutrition education programs can directly address food insecurity and improve overall health outcomes in underserved areas. - Patient Navigation Services:
These services help patients navigate the complex healthcare system, ensuring they have access to necessary medical and social resources. This can be particularly beneficial for patients facing language barriers or lacking health literacy. - Integrated Care Models:
Coordinating care between healthcare providers and social service organizations ensures that patients receive comprehensive support, addressing both medical and social needs. This model can reduce hospital readmissions and improve chronic disease management. - Policy Advocacy:
Healthcare organizations can advocate for policies that address SDOH at a systemic level, such as affordable housing initiatives, improved public transportation, and increased funding for education and healthcare services.
The Future of SDOH in Healthcare Analytics
The future of healthcare analytics is intrinsically linked to the comprehensive integration of SDOH. As data collection methods and technologies advance, our ability to capture and analyze SDOH will become increasingly sophisticated. The following trends are likely to shape the future landscape:
- 1. Real-Time Data Integration: The ability to integrate real-time SDOH data, such as economic indicators and environmental factors, will enable dynamic risk assessments and timely interventions.
- 2. Machine Learning and AI: Advanced algorithms can identify complex patterns and interactions among various SDOH, providing deeper insights into patient risk profiles and potential interventions.
- 3. Collaborative Platforms: Shared platforms that allow healthcare providers, social services, and community organizations to collaborate and share data will enhance the coordination and effectiveness of interventions.
- 4. Policy and Funding Support: Increased recognition of the importance of SDOH will drive policy changes and funding support for initiatives that address these determinants, fostering a more holistic approach to healthcare.
In conclusion, understanding and integrating Social Determinants of Health into predictive analytics is essential for the future of healthcare. By embracing the full spectrum of SDOH, providers and payers can pave the way for a healthier, more equitable future for all. The incorporation of these determinants into healthcare analytics is not just a trend but a necessary evolution to improve patient care and health outcomes.
OptMyCare specializes in leveraging AI to create actionable risk stratification solutions that incorporate both Social Determinants of Health and clinical data, helping healthcare providers and payers enhance predictive analytics and improve patient outcomes.